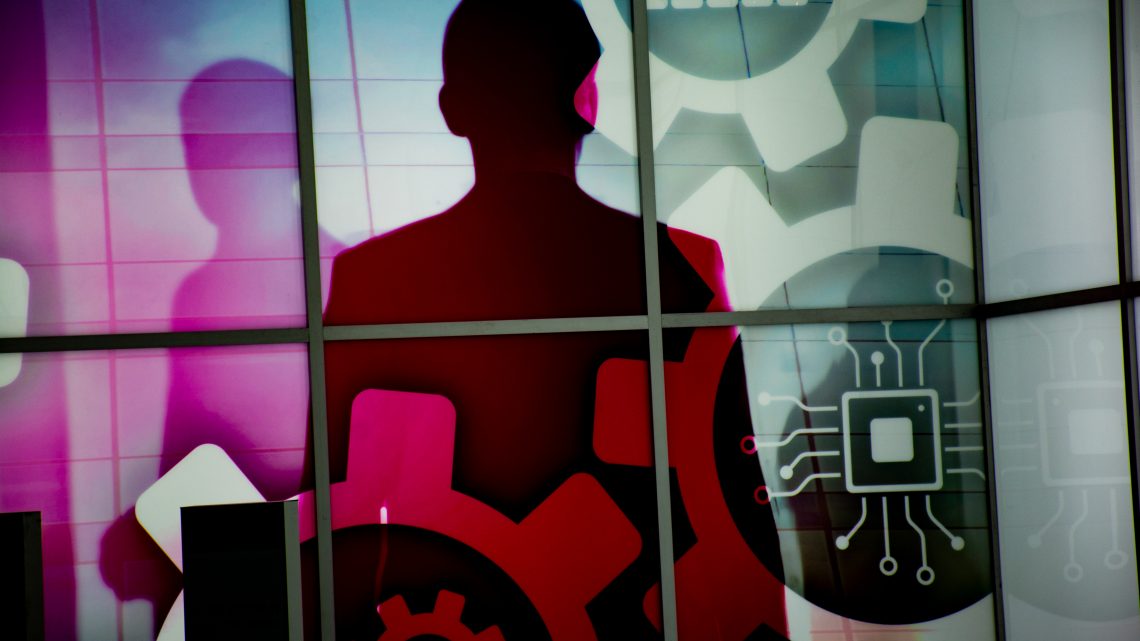
AI networks have enormous energy requirements/ MIT researchers present the solution
29. Mai 2020AI networks have enormous energy requirements/ MIT researchers present the solution
New York, 29.5.2020
Last June, researchers at the University of Massachusetts at Amherst published an astonishing report in which they estimated that the amount of energy required for training and searching in a given neural network architecture would result in the emission of about 626,000 pounds of carbon dioxide. This is nearly five times the lifetime emissions of an average US car, including its manufacture.
This problem becomes even more acute during the model introduction phase, when deep neural networks must be deployed on different hardware platforms, each with different characteristics and computing resources.
MIT researchers have developed a new automated AI system for the training and operation of certain neural networks. The results suggest that by improving the computing efficiency of the system, the system can reduce the associated carbon emissions in some key areas – in some cases to low three-digit values.
The researchers‘ system trains a large neural network consisting of many pre-trained subnetworks of different sizes that can be tailored to different hardware platforms without retraining. This dramatically reduces the energy normally required to train each specialized neural network for new platforms – which can include billions of Internet of Things (IoT) devices. Using the system to train a computer vision model, they estimated that the process would require about 1/1,300 of the carbon emissions compared to today’s state-of-the-art search approaches for neural architectures, while inference time would be reduced by a factor of 1.5 to 2.6.
„The goal is smaller, greener neural networks,“ says Song Han, assistant professor in the Department of Electrical Engineering and Computer Science. „The search for efficient neural network architectures has so far left a huge carbon footprint. But with these new methods, we have reduced that footprint by orders of magnitude“.
The work was done on Satori, an efficient computing cluster donated to MIT by IBM that can perform 2 quadrillion calculations per second. The paper will be presented next week at the International Conference on Learning Representations. Besides Han, four students and PhD students from EECS, the MIT-IBM Watson AI Lab and Shanghai Jiao Tong University are involved in the paper.
Creation of a „once for all“ network
„How do we efficiently train all these networks for such a wide range of devices – from a $10 IoT device to a $600 smartphone? Given the diversity of IoT devices, the computational cost of finding neural architectures will explode,“ says Han.
The researchers invented an AutoML system that trains only a single, large „once-for-all“ (OFA) network that serves as a „mother“ network, interleaving an extremely high number of subnets that are sparsely activated from the mother network. OFA shares all its learned weights with all subnets – i.e. they are essentially pre-trained. In this way, each subnet can work independently at inference time without retraining.